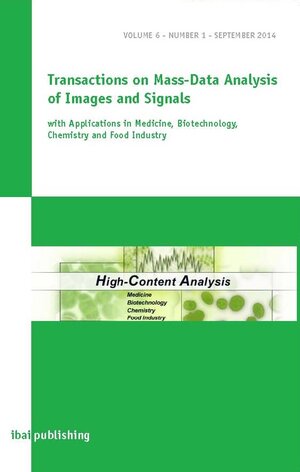
×
Wissenschaftler, Fachpublikum
Transaktions on Mass-Data Analysis of Images and Signals
with Applications in Medicine, Biotechnology, Chemistry and Food Industry
herausgegeben von Petra PernerThis journal contains two papers: one is about the analysis of anesthesia stages based on EEG entropy estimation [1] and the second one is about the observation of the internal mitochondrial movement of the cells by classification into classes round, tubular and cell death [2].
Both topics are hot topics in medicine and computational biology.
Automatic anesthesia depth analysis with the use of EEG-signal is of tremendous importance [1]. Its solution provides more precise assessment of the patient’s physio-logical condition and makes it possible to manage anesthesia process adequately. The authors propose in their paper to recognize the different stages of anesthesia depth by using the modified Kolmogorov entropy.
The observation of the internal mitochondrial movement in the cells is of impor-tance to recognize the influence of drugs or other chemical compound applied to the cells [2]. In the experiment, the biologist decided to recognize the status of the cells by three different classes: class “round”, class “tubular” and class “cell death”. These classes describe the initial, the intermediate and the end status of the internal mito-chondrial movement in the cells. In one image are more than 100 cells and the whole experiment will contain more than 10 000 images.
Automatic image processing and feature analysis procedures as well as a classifier have been selected to automatically analyze the cells in an image. The classifier is set up based on prototypical cell appearances in the image such as for e. g. „healthy cell“, „cell dead“, and „cell in transition stage“. For these prototypes are calculated image features based on random set theory that describes the texture on the cells. The prototype is represented then by the feature-value pair and the class label. These settings are taken as initial classifier settings in order to acquire the knowledge about the dynamic signatures. The approach shows impressively how for a trail-and- test application as in drug discover or computational biology where the requirement for the analysis depend on the discover-task with such an approach can easily set up an automatic image analysis and classification system in order to automatically classify thousands of cells in an image.
Both topics are hot topics in medicine and computational biology.
Automatic anesthesia depth analysis with the use of EEG-signal is of tremendous importance [1]. Its solution provides more precise assessment of the patient’s physio-logical condition and makes it possible to manage anesthesia process adequately. The authors propose in their paper to recognize the different stages of anesthesia depth by using the modified Kolmogorov entropy.
The observation of the internal mitochondrial movement in the cells is of impor-tance to recognize the influence of drugs or other chemical compound applied to the cells [2]. In the experiment, the biologist decided to recognize the status of the cells by three different classes: class “round”, class “tubular” and class “cell death”. These classes describe the initial, the intermediate and the end status of the internal mito-chondrial movement in the cells. In one image are more than 100 cells and the whole experiment will contain more than 10 000 images.
Automatic image processing and feature analysis procedures as well as a classifier have been selected to automatically analyze the cells in an image. The classifier is set up based on prototypical cell appearances in the image such as for e. g. „healthy cell“, „cell dead“, and „cell in transition stage“. For these prototypes are calculated image features based on random set theory that describes the texture on the cells. The prototype is represented then by the feature-value pair and the class label. These settings are taken as initial classifier settings in order to acquire the knowledge about the dynamic signatures. The approach shows impressively how for a trail-and- test application as in drug discover or computational biology where the requirement for the analysis depend on the discover-task with such an approach can easily set up an automatic image analysis and classification system in order to automatically classify thousands of cells in an image.