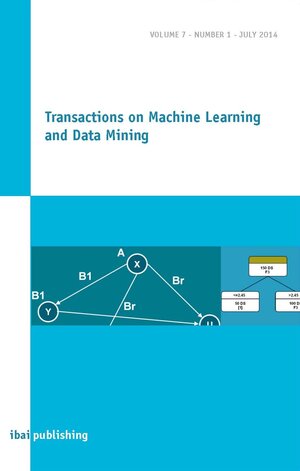
×
Wissebschaftler, Fachpublikum
Transactions on Machine Learning and Data Mining
Volume 7 - Number 1 - July 2014
herausgegeben von Petra PernerThis journal contains two papers about hot topics in the similarity-based framework. One is about similarity-based time series analysis using the shot-gun similarity [1] and the second one is about combing expert´s judge similarity with the numerical calculated similarity [2] in a similarity-based classification framework.
A new way to use the shotgun distance as similarity measure to compare time series is proposed in the first paper [1]. The introduction of the paper gives a good understanding of the shortcomings of well-known similarity measures such as the Euclidean distance and the dynamic time warping method. These measures require extracting equal-length, equal-scale, and aligned patterns from the time signal. This makes the application of these measures computational expensive since in a preprocessing step the sequences have to be preprocessed. The task often requires human interaction that makes it time-consuming and laborious expensive. The shotgun distance algorithm is further developed and carefully evaluated on different applications. The applicability of the shotgun distance in the context of hierarchical clustering of heraldic shields, and human motion detection is shown in the paper. A time series is segmented using varying lengths as part of the shotgun ensemble classifier. This classifier improves the best published accuracies on case studies in the context of bioacoustics, human motion detection, spectrographs or personalized medicine.
In the second paper [2], the expert´s judged similarity between the objects and the calculated numerical similarity of the image objects by image processing procedures is given as input to the prototype-based classifier. The prototype-based classifier is working based on prototypes the expert has been chosen. The subjective given simi-larity between the object in combination with the numerical calculated similarity helps to come close to the true similarity between the objects. The numerical calcu-lated similarity is usually influenced by the chosen attributes, the accuracy of the image procedure that calculates the attributes, and the noise in the image. This influ-ence is reduced by combining the expert´s similarity and the numerical similarity based on the least square methods to a new revised similarity value. It can be shown that the accuracy of the classifier improves while doing this. The developed approach gives a new way of using different pieces of knowledge in a similarity-based frame-work.
A new way to use the shotgun distance as similarity measure to compare time series is proposed in the first paper [1]. The introduction of the paper gives a good understanding of the shortcomings of well-known similarity measures such as the Euclidean distance and the dynamic time warping method. These measures require extracting equal-length, equal-scale, and aligned patterns from the time signal. This makes the application of these measures computational expensive since in a preprocessing step the sequences have to be preprocessed. The task often requires human interaction that makes it time-consuming and laborious expensive. The shotgun distance algorithm is further developed and carefully evaluated on different applications. The applicability of the shotgun distance in the context of hierarchical clustering of heraldic shields, and human motion detection is shown in the paper. A time series is segmented using varying lengths as part of the shotgun ensemble classifier. This classifier improves the best published accuracies on case studies in the context of bioacoustics, human motion detection, spectrographs or personalized medicine.
In the second paper [2], the expert´s judged similarity between the objects and the calculated numerical similarity of the image objects by image processing procedures is given as input to the prototype-based classifier. The prototype-based classifier is working based on prototypes the expert has been chosen. The subjective given simi-larity between the object in combination with the numerical calculated similarity helps to come close to the true similarity between the objects. The numerical calcu-lated similarity is usually influenced by the chosen attributes, the accuracy of the image procedure that calculates the attributes, and the noise in the image. This influ-ence is reduced by combining the expert´s similarity and the numerical similarity based on the least square methods to a new revised similarity value. It can be shown that the accuracy of the classifier improves while doing this. The developed approach gives a new way of using different pieces of knowledge in a similarity-based frame-work.